Exploiting Depth Information for Wildlife Monitoring
Camera traps are a proven tool in biology and specifically biodiversity research. However, camera traps including depth estimation are not widely deployed, despite providing valuable context about the scene and facilitating the automation of previously laborious manual ecological methods. In this study, we propose an automated camera trap-based approach to detect and identify animals using depth estimation. To detect and identify individual animals, we propose a novel method D-Mask R-CNN for the so-called instance segmentation which is a deep learning-based technique to detect and delineate each distinct object of interest appearing in an image or a video clip. An experimental evaluation shows the benefit of the additional depth estimation in terms of improved average precision scores of the animal detection compared to the standard approach that relies just on the image information. This novel approach was also evaluated in terms of a proof-of-concept in a zoo scenario using an RGB-D camera trap.
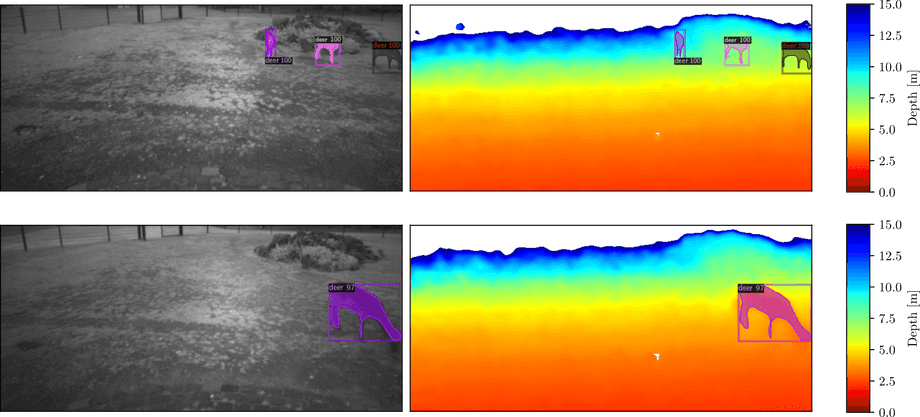
Code
We provide our synthetic data rendering code on GitHub.
Acknowledgments
We gratefully acknowledge the German Federal Ministry of Education and Research (Bundesministerium für Bildung und Forschung (BMBF), Bonn, Gemany (AMMOD - Automated Multisensor Stations for Monitoring of BioDiversity: FKZ 01LC1903B) for funding. We thank Thomas Ensch, Michael Gehlen and the entire team of the Lindenthaler Tierpark for their cooperation by hosting our experimental camera trap hardware on-site. We thank Alejandro Berni Garcia for his help with the construction of the wooden camera trap casing.
Cite
In plaintext:
Haucke, T., & Steinhage, V. (2021). Exploiting Depth Information for Wildlife Monitoring. CoRR, abs/2102.05607. https://arxiv.org/abs/2102.05607
In BibTeX:
@article{haucke2021depth,
author = {Timm Haucke and Volker Steinhage},
title = {Exploiting Depth Information for Wildlife Monitoring},
journal = {CoRR},
volume = {abs/2102.05607},
year = {2021},
url = {https://arxiv.org/abs/2102.05607},
eprinttype = {arXiv},
eprint = {2102.05607},
timestamp = {Thu, 18 Feb 2021 15:26:00 +0100},
biburl = {https://dblp.org/rec/journals/corr/abs-2102-05607.bib},
bibsource = {dblp computer science bibliography, https://dblp.org}
}